Amazon and Johns Hopkins University (JHU) today announced the second-year recipients of PhD fellowships and faculty research awards as part of the JHU + Amazon Initiative for Interactive AI (AI2AI).
The AI2AI initiative, launched in April 2022 and housed in JHU’s Whiting School of Engineering, is focused on driving ground-breaking AI advances with an emphasis on machine learning, computer vision, natural-language understanding, and speech processing.
As part of the initiative, annual Amazon fellowships are awarded to PhD students enrolled in the Whiting School of Engineering. Amazon also funds research projects led by JHU faculty in collaboration with post-doctoral researchers, undergraduate and graduate students, and research staff.
Below is a list of the awarded fellows and their research projects, followed by the faculty award recipients and their research projects.
Academic Fellow Recipients
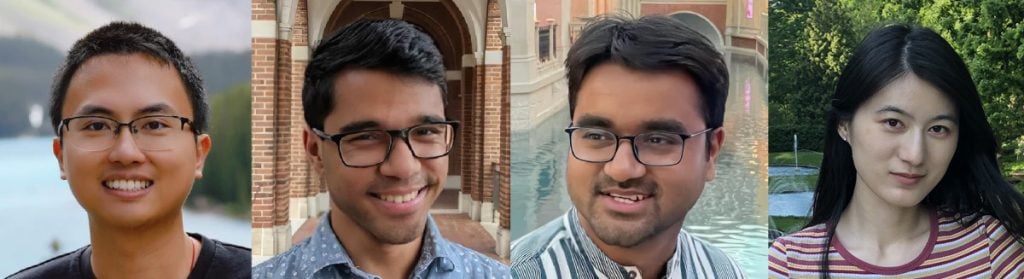
From left to right: Jiang Liu, Ambar Pal, Aniket Roy, and Xuan Zhang.
Jiang Liu is a fifth-year PhD student studying electrical and computer engineering and advised by Rama Chellappa. His research is focused on developing responsible and trustworthy AI systems, including computer vision algorithms that are robust to adversarial attacks, facial-privacy protection techniques, and multimodal AI algorithms that can understand both vision and language.
Ambar Pal is a final-year PhD student studying computer science and advised by René Vidal and Jeremias Sulam. He is focused on the theory and practice of safety in AI, with a central philosophy that incorporating structural constraints from data can efficiently mitigate vulnerabilities to malicious agents in current ML systems.
Aniket Roy is a fourth-year PhD student studying computer science under the guidance of Chellappa. He is researching computer vision and machine learning — specifically, few-shot learning, multimodal learning, and generative AI, including diffusion models and large language models.
Xuan Zhang is a fifth-year PhD student studying computer science under the guidance of Kevin Duh. She is focused on sign language processing, with an emphasis on sign language recognition and translation.
Faculty Recipients
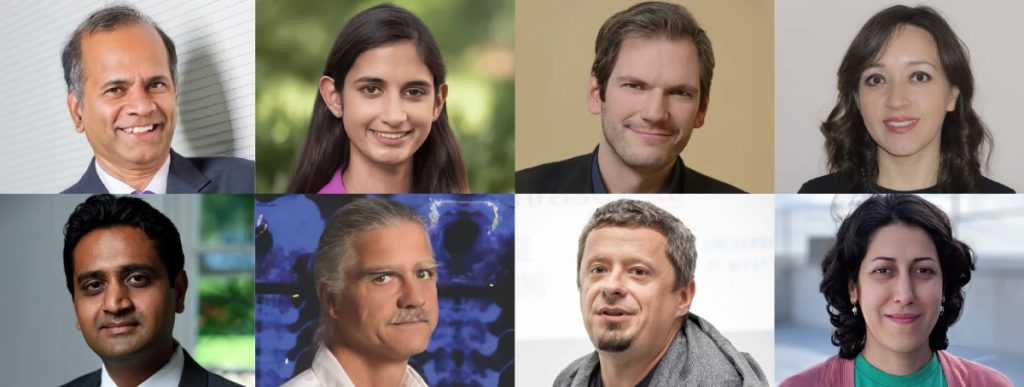
Top row, left to right: Rama Chellappa, Anjalie Field, Philipp Koehn, and Leibny Paola Garcia Perera; second row, left to right: Vishal Patel, Carey Priebe, Jan Trmal, and Masha Yarmohammadi.
Rama Chellappa, Bloomberg Distinguished Professor in the department of electrical and computer engineering and the department of biomedical engineering: “Self-supervision for skeleton-based learning of actions”
“Supervised learning of skeleton sequence encoders for action recognition has received significant attention. However, learning such encoders without labels continues to be a challenging problem. In this work, we propose to build on a contrastive-learning approach developed during the first year of the AI2AI effort. We will collaborate with Amazon researchers on further hardening the proposed approach and test on real-life sequences to validate its effectiveness and robustness.”
Anjalie Field, assistant professor of computer science: “Fair and private NLP for high-risk data”
“This proposal aims at developing text generation tools to create realistic synthetic data that can facilitate research and model development while improving model fairness and minimizing privacy violations.”
Philipp Koehn, professor of computer science: “Convergence of language and translation models”
“We propose to combine the strengths of both large language models (LLMs) and neural machine translation, especially the ability of LLMs to model wider, multi-sentence context and larger amounts of training data and translation models’ focus on the actual task in a supervised way.”
Leibny Paola Garcia Perera, assistant research scientist: “On-device compressed models for speaker diarization”
“In this proposal, we will study how to build efficient diarization models based on self-supervised models that can be deployed on-device.”
Vishal Patel, associate professor in the Vision & Image Understanding (VIU) Lab: “Language-guided universal domain adaptation”
“Recent advances in deep learning have led to the development of accurate and efficient models for various computer vision applications such as classification, segmentation, and detection. However, learning highly accurate models relies on the availability of large-scale annotated datasets. As a result, these approaches suffer from severe degradation of performance when evaluated on images that are sampled from a different distribution than that of training images. To overcome these issues, we propose to develop a vision-language model-guided universal domain adaptation method, which aims to handle both domain shift and label shift between domains in the wild.”
Carey Priebe, professor, department of applied mathematics and statistics, and director, Mathematical Institute for Data Science (MINDS): “Comparing large language models using data kernels”
“We propose a framework for comparing and contrasting the representation spaces of deep neural networks — specifically, large language models (LLMs) before and after the introduction of reinforcement learning from human feedback (RLHF) — that is simultaneously computationally practical, statistically principled, mathematically tractable, and visually interpretable.”
Jan Trmal, associate research scientist, Center for Language and Speech Processing (CLSP), and Masha Yarmohammadi, assistant research scientist, CLSP: “Developing an evaluation protocol for contextualized ASR”
“We propose to develop an evaluation protocol that has a wide variety of types of scenarios in which speaker context information can be incorporated into the recognition process.”
(This article originally appeared on Amazon Science)
The post Johns Hopkins and Amazon Announce Four Fellow and Eight Faculty Research Awards appeared first on Johns Hopkins Whiting School of Engineering.